Automating data governance: 5 benefits of modern data mapping
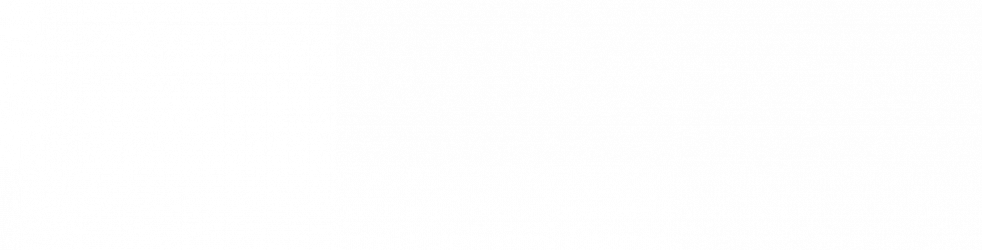
Data privacy legislation like GDPR and CCPA has caused a certain amount of upheaval among businesses.
When GDPR went into effect, many organizations weren’t in compliance, and some still weren’t sure where to start. With the advent of CCPA in California, and ongoing talks at the FTC about national consumer data privacy rules for the U.S., the issue of data governance will only grow more visible and more critical.
Unfortunately, many businesses still aren’t ready. Many have no documentation on how data moves through their organization, how it’s modified, or where it’s stored. Some attempt documentation using spreadsheets that lack version control and are at the mercy of human error.
But the implications of data governance go well beyond compliance. Here are five reasons why it’s time to professionalize your documentation and map your data using automation.
1. Compliance
Data privacy laws are arriving whether businesses like it or not. GDPR famously caught businesses by surprise, despite a two-year heads-up that it would be taking effect. Less than a month before GDPR was set to take effect in May 2018, Gartner predicted that more than half of companies affected by the law would fail to reach compliance by the end of the year. More than 18 months later, at the end of 2019, new data suggested 58% of GDPR-relevant companies still couldn’t address data requests in the designated time frame.
To achieve compliance with GDPR and CCPA, you essentially need to know how data comes into your company, where it goes, and how it’s transformed along the way. Organizations struggle to do so because they haven’t properly mapped data’s path through their environment.
Spreadsheets are no solution. To prove compliance, you need accurate, current, and centralized documentation mapping your data. Automated tools speed up the process and deliver foolproof compliance.
2. Saving time and resources
Data scientists bring a lot of value to an organization, but given their specialized and in-demand skills, the average base salary for a data scientist ranges from $113,000 to $123,000. More experienced data scientists command even more.
Unfortunately, at many organizations, data scientists spend 30-40% of their time doing data preparation and grooming, figuring out where data elements came from, and other basic tasks that could be automated.
When data scientists spend so much time on basic tasks, the organization isn’t just losing the time and cost it takes to do that work, it’s losing the opportunities that could be uncovered if the data scientists spent more of their time on data modeling, actionable insights, and predictive analysis.
3. Accelerating modernization efforts
Many companies are looking to transition their data from in-house data centers to more cost-efficient cloud databases, where they only pay for the compute power they use. It’s an opportunity to realize cost savings and modernize their environment.
But it can be a strenuous process if you don’t know what’s flowing into on-premises hardware, because you won’t be able to point the same pathways at the cloud. Documenting those pathways manually can be precarious and time-consuming at best. Automated tools that map the data pathways for you can accelerate the transition.
4. Improving communication and collaboration between business and IT
Business and IT often live in different worlds. This is one of the reasons why so few companies have successfully mapped their data. The ETL coders live in the world of data, so when asked about mapping, they just say it’s in the code. They’ve been trained to prioritize productivity in their code, not to focus on documenting, and that different mindset can create a disconnect with business users.
Business users can see IT as uncooperative. IT can see business users as demanding. But with the right tools, you can take this area of contention and turn it into business value.
With the right tools, you can generate glossaries relating business terms to technical metadata and find every term related to GDPR, for example, offering business users the insight they need. IT can do what it has been trained to do, and business users can access data lineage and even a whole architectural overview. The end result is better communication and collaboration between the two.
5. Making confident and precise decisions
If you haven’t mapped your data, if you don’t know where the information came from, how it was modified, and so on, that creates major problems for any actionable insights you’ve extracted from the data. If you don’t know where the data originated, how can you trust that it’s accurate and a solid basis for decision-making?
Without that certainty, you might have decisions that are directionally correct, but lack precision. With detail, transformation logic, and comprehensive documentation, you can trust the data is high quality and accurate, and any decisions based on that data are more targeted and precise.
Automating data mapping and governance
Especially now that major consumer privacy laws are active and others are taking shape, it’s essential to know the entire path of data through your organization and to map and understand that path.
But manual processes, no processes, and spreadsheets won’t cut it anymore. All are dated strategies from years ago, before the explosion in data collection, before user-friendly automated tools widely existed.
Asking ETL coders to document what they’re coding is often a battle you’ll lose. But ultimately, you don’t need to ask that question. Automated tools can map the path of data and provide full documentation of your data lineage and impact analysis that’s scalable, always up to date, and not vulnerable to human error.
Data governance is more important than ever. It’s time to invest in the tools to prioritize it.
About the author
Sam Benedict serves as Vice President – Solution Strategy at erwin. He is responsible for helping clients and partners understand the value of erwin’s EDGE Platform, aligning their needs with the many features and functions to solve problems and create sustainable data governance programs. With more than 25 years in technology, Sam is a strategic planner around enterprise data management, data governance, and system development lifecycle improvement. Sam has worked in the telecommunications, healthcare, and manufacturing industries, globally, and understands the challenges faced by IT resources, as well as business stakeholders in the information management space.